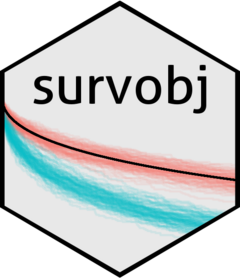
Simulation of survival times
Source:vignettes/simulation_distributions.Rmd
simulation_distributions.Rmd
Introduction
Following Bender, Augustin, and Blettner
(2003) and Leemis (1987),
simulation of survival times is possible if there is function that
invert the cumulative hazard
(),
Random survival times for a baseline distribution can be generated from
an uniform distribution between 0-1
as:
For a survival distribution object, this can be accomplished with the
function rsurv(s_object, n)
which will generate
n
number of random draws from the distribution
s_object
. All objects of the s_distribution family
implements a function that inverts the survival time with the function
invCum_Hfx()
The function ggplot_survival_random()
helps to graph
Kaplan-Meier graphs and cumulative hazard of simulated times from the
distribution
s_obj <- s_exponential(fail = 0.4, t = 2)
ggplot_survival_random(s_obj, timeto =2, subjects = 1000, nsim= 10, alpha = 0.3)
Generation of Proportional Hazard times
Survival times with hazard proportional to the baseline hazard can be simulated where is a hazard ratio.
The function rsurv_hr(s_object, hr)
can generate random
number with hazards proportionals to the baseline hazard. The function
produce as many numbers as the length of the hr vector. for example:
s_obj <- s_exponential(fail = 0.4, t = 2)
group <- c(rep(0,500), rep(1,500))
hr_vector <- c(rep(1,500),rep(2,500))
times <- rsurvhr(s_obj, hr_vector)
plot(survfit(Surv(times)~group), xlim=c(0,5))
The function
ggplot_survival_hr()
can plot simulated data
under proportional hazard assumption.
s_obj <- s_exponential(fail = 0.4, t = 2)
ggplot_survival_hr(s_obj, hr = 2, nsim = 10, subjects = 1000, timeto = 5)
Generation of Acceleration Failure Times
Survival times with accelerated failure time to the baseline hazard can be simulated where is a acceleration factor, meaning for example an AFT of 2 have events two times quicker than the baseline
The function rsurv_aft(s_object, aft)
can generate
random numbers accelerated by an AFT factor. The function produce as
many numbers as the length of the aft vector. for example:
s_obj <- s_lognormal(scale = 2, shape = 0.5)
ggplot_survival_aft(s_obj, aft = 2, nsim = 10, subjects = 1000, timeto = 5)
In this example, the scale parameter of the Log-Normal distribution represents the mean time and it this simulation and accelerated factor of 2 move the average median from 2 to 1
If the proportional hazard and the accelerated failure is combined
and accelerated hazard time is generated. This can be accomplished with
the function rsurvah()
function and the
ggplot_random_ah()
functions